Case studies
Proven results, right away
Trusted to identify the best data solutions
With our experience and expertise, we deliver wins immediately
20+
Digital Transformations
20+
Data Platforms
35+
Data Warehouse
25+
MLOps/DataOps
50+
Database Migrations
14+
Years in Data Analytics Modernization
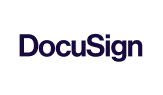
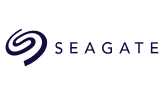
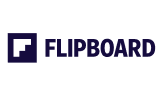
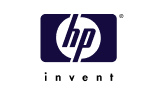
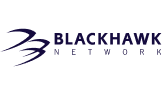
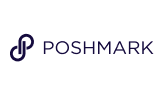
“We’re more resilient, and Flipboard’s overall user engagement has improved as a direct result of this migration.”
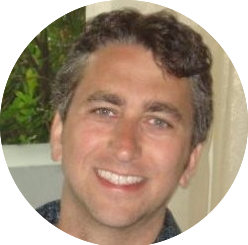
Greg Scallan
VP of Engineering, Flipboard
“We were able to optimize and confidently re-architect our HPC Clusters for better resource utilization, enhanced job performance, improve the throughput of one of our largest EDA clusters by up to 40%, and improve queue contention related to jobs having 75% lesser ‘wait/pending’ times using smart queues. The AI-driven forecasting model will help us predict future resource requirements and accelerate our cloud journey.”

Michael Brooker
CIO, Synaptics
“A big thank you to the Mactores team for the great execution! As a company, Poshmark recognizes how complex this project was, as well as all the hard work it took to make this a smooth migration. Great team effort!”

Gautam Golwala
Co-founder and CTO, Poshmark